It is critical in both your traditional and AI-driven data analytics to take proactive steps to “characterize” your data supply chain.
In this article, we describe how data supply chains operate and the need to differentiate your data on quality, reliability, security, and price.
You’ll learn how Zectonal monitors data supply chains in a unique way by focusing on characterizing the contents of the most fundamental component – the data itself.
Unlike other data managements tools that focus on monitoring systems in a piecemeal fashion or allow you to ask ambiguous questions of your data, Zectonal monitors the actual content of your data, providing uniquely insightful quality metrics and answers about the data you need to operate your data-driven enterprise.
Zectonal also protects and secures your data supply chain by looking for advanced threats inserted into the global data supply chain with unknown origin, and with the potential to poison and severely disrupt your AI analytic products.
Defining Components of a Data Supply Chain
We define 5 data supply chain components using the analogy of a basic physical-parts supply chain which facilitated economic development for most of the Industrial Age.
AI-Generated Analytic Products = Traditional Finished Goods
In physical supply chains, there is a finished good such as a car or a widget created to increase the wellbeing of society or improve our daily lives. In the data supply chain, the finished good is a data analytic product, increasingly created through the use of AI. These analytic products allow organizations to differentiate themselves for competitive advantage.
Software Analytic and AI tools =Traditional Manufacturing Equipment
Open source and proprietary software including Big Data distributed systems, cloud storage, and now AI platforms are akin to the manufacturing equipment and tooling that organizations invest in, configure, and operate to generate their unique analytic insights.
Data Lake = Traditional Manufacturing Facilities
The Data Lake and Data Warehouse, or some variant thereof, are equivalent to the traditional manufacturing facility. The facility is no longer physical (it is in software), but a virtual location where data is collected, organized, and secured for an organization’s use.
Data Pipelines =Traditional Transportation Fleets
To move the data its source, either its original creation of some other intermediary processing location, the data supply chain relies on the data pipeline to ensure an efficient, secure, and sometimes near real-time delivery from external localities.
Data = Traditional Raw Ingredients
Much like the raw physical components of physical supply chains, data is the raw ingredient and can take many forms.
“In the earliest days of the automobile, Henry Ford made a decision to own or control the entire supply chain—from the mines that provided the ore to the factories that made the glass. Raw materials—iron ore, coal, and rubber, all from Ford-owned mines and plantations—came in through one set of gates at the plant while finished cars rolled out the other. Today it is exceptionally rare for a company to try to own all the raw materials for a physical product. Even software products use pre-existing software frameworks and code.[1]”

It is virtually impossible to accomplish what Henry Ford did by controlling every aspect of a data supply chain in modern times. Modern enterprises will be reliant on opaque data supply chains whose tiers and origins are not fully understood. As Henry Ford’s business matured and become more complex, his original supply chain strategy has changed quite a bit over the past 100 years.
More than 100 years after Henry Ford controlled the entire supply chain for his cars, by 2020 it was estimated Ford’s supply chain contains more than 1,400 Tier 1 suppliers and up to 10 tiers between supply and raw materials.[2]
In this next section, we describe a data supply chain using weather data. The weather data supply chain is used as an example since it is useful for almost every type of industry and firm. It is also easy to relate to the information this supply chain contains, and as humans, we have a fundamental understanding of the analytic predictions it can produce. Weather data is easy to understand as it can contain hundreds of attributes we can all comprehend including temperature, humidity, pressure, etc. Other types of data are more esoteric, with opaque relationships at various tiers of the supply chain.
Your Data Supply Chains Are An Aggregation of Smaller Data Supply Chains
An organization’s Tier-1 data suppliers are the external third-party companies where you directly procure data for your enterprise. Weather data is commonly used by the retail industry, hospitality industry, transportation industry, and even the financial industry. Fluctuations in weather even have some correlation to movements in stock prices. Unlike Henry Ford’s original supply chain, it is very unlikely in this day and age that a single company owns and operates, at any reasonable scale, a network of thermometers that produce weather data. Guess what – these Tier-1 weather data providers rarely own the thermostats and equipment to generate the raw weather data either! Below is an example of the NWS soliciting individual weather stations to provide data as part of their data supply chain.
Do you own a personal home weather station and a computer with a dedicated connection to the Internet, such as fiber, DSL or cable? If you do, the National Weather Service (NWS) and local television meteorologists would love to see your data!
https://www.weather.gov/iln/cwop
How Are Data Supply Chains Differentiated?
Data supply chains and the products created from this data should be differentiated or characterized in the same way we measure traditional supply chains.
- Quality – Do the sourced items meet a mutually agreed-upon specified quality standard that will allow me to create differentiated products quickly and with minimal resources?
- Reliability – Can I reliably obtain sourced items in a consistent and expected manner?
- Security – Can I reasonably expect the procured items will be free of intentional or unintentional defects that could harm the manufacturing equipment and result in fault products?
- Price – Am I paying a fair price based on the quality and reliability of the data I procured?
What’s the Problem, Then?
The problem is:
- Almost no organization measures the data they procure based on agreed-upon quality standards
- Very few organizations negotiate data availability in the form of Service Level Agreements (“SLA’s”)
- Security usually only extends to software systems and not the actual content or quality of the data itself
- For the benefit of most data providers, the price of data usually has no bearing other than what a good salesperson can extract from a less-experienced buyer
On this last point around pricing, this is why hedge funds often pay exorbitant salaries for individuals responsible for purchasing their commercial data since their personal experiences, rather than industry quality standards, are the only way to quantify what constitutes a fair price.
Disruption Risks With Data Supply Chains
Similar to physical supply chains, it is not uncommon for individual suppliers deep within the supply chain to intentionally or unintentionally introduce quality defects to their upstream consumers. We refer to this as Data Poisoning. We have found intentional defects are introduced when a data supplier is copying the same data over and over again in order to appear as though the data volumes they are generating are consistent. Data volume, a misleading characteristic by itself, is one way data providers price and sell their resources. Since the volumes are consistent, these type of “cheap knockoff’s” can go unnoticed for a long time while still getting paid. Combatting this requires the content of the data to be monitored for duplicity.
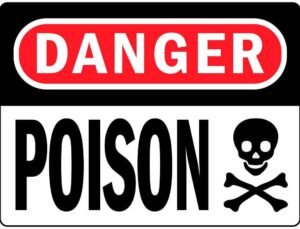
Unintentional defects often arise when the machinery, (i.e. the software analytic tools, and the associated Extract Transform Load “ETL” jobs) introduce flaws based on data schema changes (too many or too few columns or rows) and data types (integer versus float versus string, etc.). If a data supply chain is non-trivial, detecting these data quality defects becomes extremely challenging. Monitoring the contents of the data for specific quality metrics, as Zectonal does, is the method for detecting these type of issues and addressing them head-on.
As we have seen recently with many physical supply chains globally most notably in the semiconductor industry, they can be disrupted, and those disruptions have far ranging consequences that are not easy to predict in advance.
Data Supply Chains Are Disrupted All The Time – You Just Don’t Know It
Once you start monitoring data supply chains, it’s actually alarming how much they do go down! Without monitoring data, disruptions go unnoticed until something bad happens, usually stemming from an erroneous prediction.
For example, it is common for weather data providers to source their Tier-1 data providers by region (country, state, locality, etc.). Those aggregators might have sub-regional providers, and so on down to individuals or neighborhood collectives. If a data aggregator suddenly stops producing data due to an operational outage, and the overall percentage of missing data for the entire aggregate weather data is small, without proper monitoring, this outage will not be detected until an erroneous insight is observed. By the time a human observes such an erroneous insight, it’s often already too late and has lead to more catastrophic consequences. Imagine weather data for a portion of Alaska goes down for a period of several weeks representing 1-2% of the total weather data for the United States. Without Zectonal’s monitoring, you might not detect such a small variance and understand the impact until you were to see an AI trained model generating a prediction for a 12-hour sunny day in the 80’s in the middle of an Alaskan winter. Since this model lacked sufficient and necessary training data, the algorithm itself worked as intended. Monitoring the health of the AI algorithm will not tell us much about what’s going on, but we know it is flawed based on our own human intuition.
For weather data and faulty weather predictions, our human intuition can provide a reasonable sanity check. A more dangerous scenario is what if our Alaska forecast was only off by a few minutes or hours of sun, or only a few degrees of temperature? Would human intuition detect it then? The answer is no.
For most other data sets for which human’s have no inherent intuitive knowledge of, we need software to perform those sanity checks. That’s where Zectonal comes into play.
Zectonal Removes Risk From Your Data Supply Chains
As we described previously in our article 7 Reasons Why DataOps Monitoring Will Improve Your Analytics. Those same reasons are relevant here as well.
Not all data will be high quality or have a high reliability standard – those characteristics determine what constitutes a fair price. Sometimes it makes sense to buy low quality and unreliable data at a low cost. It never makes sense to buy it at a high cost. But what constitutes low or high quality and reliability, and what is a fair price, really? Characteristics and metrics about data quality and reliability are critical to understanding the value of data you procure, or the data you produce.
Zectonal Can Assist in Multiple Ways
Zectonal Quality Monitoring – quality data is important for training AI machine learning models and Zectonal’s Data Observability monitoring capabilities reduce friction for data scientists to understand your data quickly. AI bias is an unintentional outcome that can result from ML training data drifting from an expected baseline. Zectonal’s monitoring helps data scientists understand drift and its impact on biased predictions.
Zectonal Data SLA Monitoring – Reliable data allows AI inferencing to operate efficiently. Most organizations have no way of detecting if a portion of your data isn’t flowing, even if the algorithms are still producing predictions. Zectonal can alert you when even a small portion of the data stops flowing, providing enhanced situational awareness about your AI.
We believe data should have a reasonable expectation around security so as not to degrade or impair software analytic tools (the machinery) or bias and poison AI outcomes (the product).
Zectonal Payload Scanning and Detection – AI Poisoning and Data Poisoning are emerging cybersecurity threats resulting from intentional ML training data manipulation. Zectonal monitors the contents of data for these emerging threats.
Zectonal is the first to develop unparalleled software capabilities to ensure a complete and blazingly fast situational-awareness of your data. Feel more confident in your data. Generate impactful insights. Make better business decisions with less hassle, and at a faster pace.
Join The Conversation at Zectonal for additional Data Observability and AI Security topics in the future, including Data Supply Chain Threat Detection.
Know your data with Zectonal. https://www.zectonal.com
About the Author: Dave Hirko is the Founder and CEO of Zectonal. Dave previously worked at Amazon Web Services (AWS), Gartner, and was a Founder and PCM Member of the top-level Apache Metron Big Data Cybersecurity Platform that was implemented by Fortune 500 institutions to find cyber anomalies using Big Data analytics.
Feel free to reach out to Dave at dave@zectonal.com
[1] https://courses.lumenlearning.com/marketing-spring2016/chapter/reading-components-of-a-supply-chain/
[2] https://www.thomasnet.com/insights/ford-supply-chain/#:~:text=Creating%20Efficiencies,protect%20against%20changeable%20economic%20conditions.